Recently, Professor Chen Bin from the College of Atmospheric Sciences at Lanzhou University guided his students to publish a research article titled 'Synergistic observation of FY-4A&4B to estimate CO concentration in China: Combining interpretable machine learning to review the influencing mechanisms of CO variations' in the international geoscience journal npj Climate and Atmospheric Science. This study is based on the data of the new generation of domestically produced geostationary Fengyun-4 binary satellites (FY-4A and FY-4B) in China, and constructs an interpretable machine learning model to generate near surface CO concentrations with full coverage and high spatiotemporal resolution in China (resolution: hourly and 0.04 °); Furthermore, using this set of data, a detailed evaluation of CO pollution at the urban scale was conducted, and the causes of diurnal differences in CO were analyzed in depth, revealing important factors and possible mechanisms that affect CO.
CO poses serious risks to human health and the environment. Research on the fine scale distribution and pollution status of CO is limited, and the key factors and mechanisms affecting CO are not clear. One of the reasons is the lack of high-quality CO data with high spatiotemporal resolution. To address this scientific issue, a geostationary satellite dual satellite collaborative observation model is constructed by coupling the atmospheric top radiation data of FY-4A and FY-4B; Compared to using a clear sky dataset (cloud removal model), the model using an all day dataset (cloud retention model) improved by 6.6%; The retained cloud area model can not only achieve full coverage estimation of near surface CO concentration, but also has higher estimation accuracy, indicating that the constructed machine learning model has strong big data learning and prediction capabilities. Research has found that the daily variation of CO concentration is mainly influenced by local emissions and meteorological conditions. However, in certain regions (such as the Qaidam Basin), human activities are limited, and terrain factors lead to rapid diffusion of CO. The daily variation of CO pollution levels in different regions shows a decreasing trend from north to south, with the greater the difference, the higher the degree of pollution. The study also shows that there is a significant difference in CO between day and night. In most parts of China, the concentration of CO at night is slightly higher than during the day, mainly related to the diffusion and absorption conditions of pollutants at night.In most regions, the weakening of atmospheric circulation and the decrease in planetary boundary layer height lead to the accumulation of near surface CO, resulting in higher nighttime CO concentrations. In some areas with lush vegetation coverage (such as the southeast coast, southern Yunnan, southern Xizang and northeast China), pollution can be effectively reduced through dry deposition, and CO has a lower concentration at night. Regions with lower nighttime CO concentrations than during the day are mainly distributed in areas with lower emissions, better diffusion conditions, and higher vegetation coverage. In order to explore the key factors affecting CO, a machine learning model interpretability analysis was conducted by combining feature importance and SHAP method. The global interpretability analysis of the SHAP algorithm is similar to the feature importance of the model, with meteorological factors contributing the most to CO changes. When conducting SHAP analysis on daytime and nighttime datasets, the importance of meteorological factors increased by 51% during the day, indicating that the difference in CO levels between daytime and nighttime is largely influenced by meteorological factors, while nighttime CO levels are more influenced by factors such as altitude and vegetation cover. This study can provide basic data and scientific support for China's 'pollution reduction and carbon reduction' strategic goals.
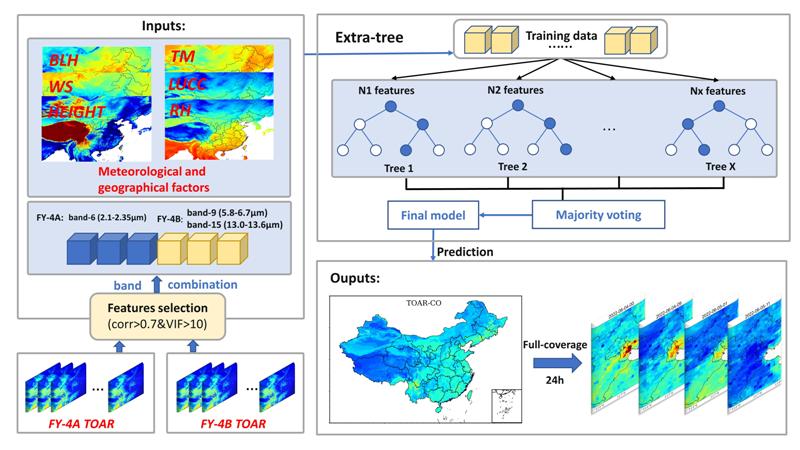
Figure 1: Framework diagram of CO machine learning model for FY-4A and FY-4B dual star collaborative construction
Figure 2: (a) Daily variation of CO concentration in different regions (Beijing time), (b) Distribution of estimated differences in CO concentration between nighttime and daytime (nighttime concentration minus daytime concentration), (c) Characteristic importance of different variables in extreme tree (ET) model, (d) SHAP importance score based on ET model, (e) Local interpretation of specific samples based on ET model.
Professor Chen Bin is the first and corresponding author of the research paper, and his master's students Hu Jiashun and Wang Yixuan are the second and third authors, respectively. This research work has been supported by projects such as the Second Qinghai Tibet Plateau Scientific Expedition and Research Program and the Excellent Youth Support Program of Lanzhou University, a central university.